Boning Yu / Risk, Learning Conditional Probability, and Deliberation
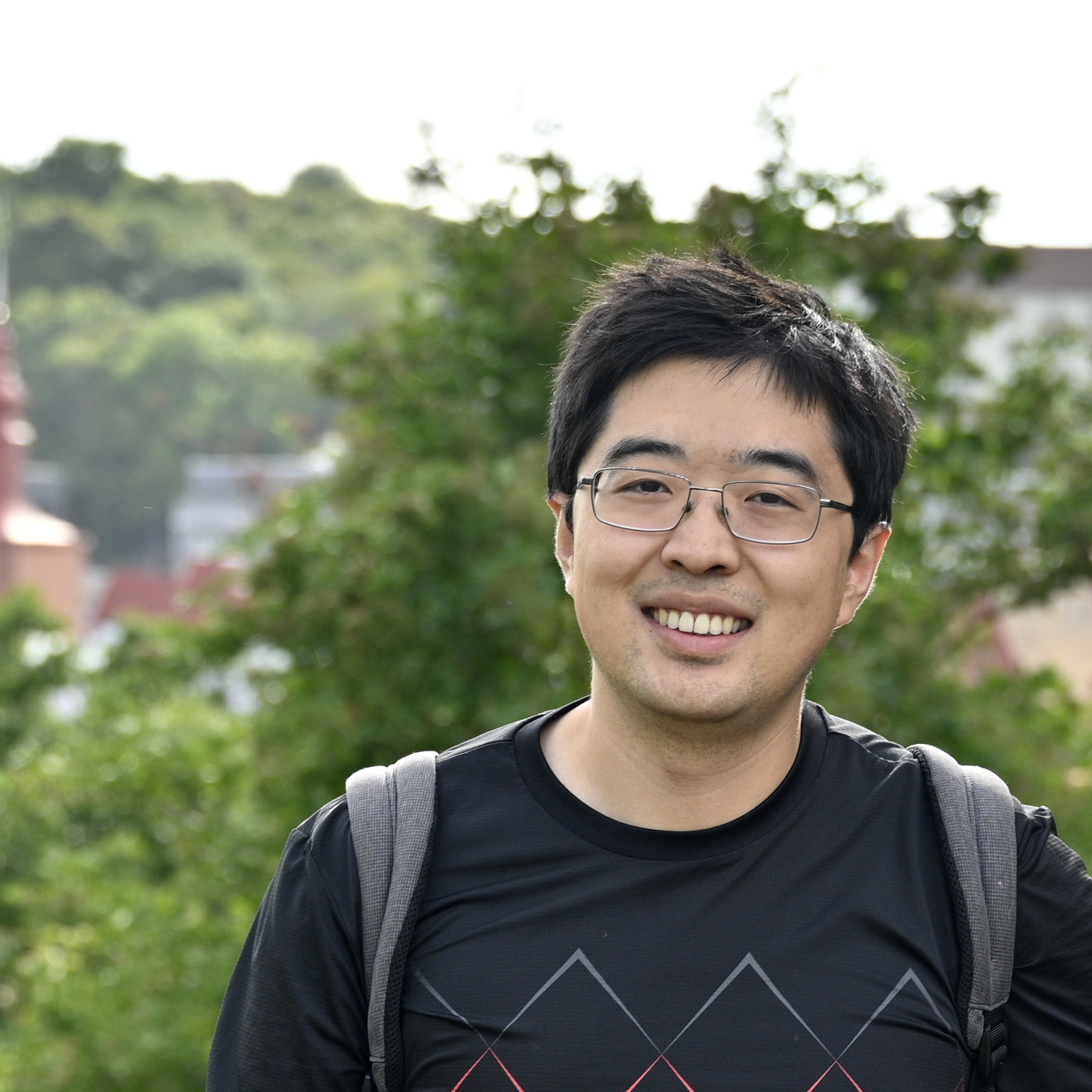
Boning Yu / Risk, Learning Conditional Probability, and Deliberation
Wednesday April 9, Boning Yu defends his dissertation, "Risk, Learning Conditional Probability, and Deliberation," abstracted below. Chaired by Eric Pacuit, the committee also includes Fabrizio Cariani, Paolo Santorio, Harjit Bhogal and, representing the Dean, Wedad Elmaghraby from the Smith School of Business.
My dissertation centers on Bayesian epistemology and its application at both individual and group levels. At the individual level, my research addresses rational decision-making under risk as well as belief updating with respect to evidence about conditionals. At the group level, my research addresses how group communication and deliberation influence a group’s ability to track the truth. In Chapter 1 A Problem of Risk-Weighted Expected Utility Theory, I prove a robust impossibility result for risk-weighted expected utility theory (REU). Using only widely accepted assumptions, I show that REU—what many consider a promising alternative to expected utility theory (EU)—necessarily falls into the same kind of failure as EU does. In Chapter 2 A Bayesian Reflection of the Judy Benjamin Problem, I investigate two Bayesian solutions to the Judy Benjamin problem and introduce a general Bayesian framework that accommodates situations that lack an obvious probability space. One solution I investigate, called the intuitive solution, aspires to but sadly fails to capture all the relevant intuitions. I suggest a superior descriptive model that can capture all the relevant institutions in a situation like the Judy Benjamin problem. In Chapter 3 Deliberation Under Dynamic Discovery of Evidence, I propose an agent-based model with two key innovations. First, it dynamically accounts for agents’ improvement in evidence-gathering ability over time. Second, my model captures two types of communication: exchanging evidence and exchanging results. Through simulations, I identify a heuristic strategy. In challenging situations, the best strategy is to learn all asserted conclusions without exchanging evidence; however, in scenarios where agents have decent access to high-quality evidence, combining full communication about conclusions with some degree of communication about evidence is the best practice.